A/B Testing: The Science Behind the Numbers
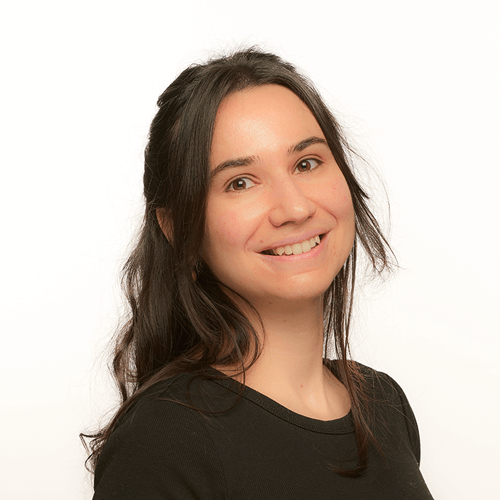
Ozlem Senlik Staff Data Scientist Airship
In the fast-paced world of digital marketing, success depends on far more than snappy slogans and eye-catching content. Behind the scenes, marketers rely on cold, hard data and statistical analysis to fine-tune their strategies and boost performance. Enter A/B testing — the bread and butter of digital marketing experiments.
But before diving into the nitty-gritty of A/B testing, it’s crucial for anyone running an experiment to answer some burning questions. How confident do we need to be with the test results? What’s the wiggle room for errors? And most importantly, how big should the differences between our variations be before implementing the winner?
Why are these questions so important? If a new variant is declared a winner and implemented without sufficient evidence of its long-term impact, it could result in significant financial setbacks. In high-stakes scenarios, prioritizing higher confidence levels, even at the expense of extending the experiment or increasing the sample size, becomes crucial. Conversely, when the risks are lower, you might opt to lower your confidence threshold, allowing for faster and more iterative experimentation to capture smaller gains promptly.
Now, let’s break down the statistical concepts behind these questions. You don’t need to be a math wiz to run successful A/B tests, but it is important you understand the statistical concepts behind the burning questions. Buckle up!
Statistical Significance
Statistical significance acts as your reliable stamp of approval — it confirms that your observations aren’t merely coincidence.
Imagine you’re analyzing the results of an A/B test comparing two versions of a landing page to see which generates more sign-ups. Achieving statistical significance means you can confidently assert, “Yes, these differences we’re seeing in user engagement are real.” Without it, you’d be navigating murky waters, making decisions based on gut feelings rather than hard data. Whether your test result reaches statistical significance depends on how other statistical metrics, such as the significance level and p-value, compare to each other.
Practical Significance vs. Statistical Significance
Practical significance is like the real-world impact of our findings — it’s what distinguishes meaningful insights from mere statistical noise.
Consider you’re conducting an A/B test on two different ad creatives to determine which generates higher click-through rates. While statistical significance may indicate that one ad outperforms the other, practical significance considers whether this difference in performance translates into tangible business benefits, such as increased sales or brand awareness. So, while statistical significance assures us of the reliability of our findings, practical significance evaluates their significance in achieving overarching marketing objectives.
P-value
P-value serves as a crucial clue — it provides insight into the likelihood that our results are merely a coincidence. Think of it as a detective’s lead, guiding us in determining the authenticity of our findings. A lower p-value suggests that our observed outcomes are unlikely to occur by chance alone, akin to having Sherlock Holmes by our side, assisting us in distinguishing truth from randomness. This value is determined through various statistical tests like the Z-test, T-test, or Chi-Square Test. Let’s delve into an example:
Suppose you’re running an A/B test to compare two different versions of an email campaign. You’re analyzing the click-through rates to determine which version performs better. After conducting the test, you calculate the p-value and find it to be 0.03.
Now, this low p-value indicates that there’s only a 3% chance that the observed difference in click-through rates between the two versions occurred due to random variability. In other words, it’s highly unlikely that the differences you’re seeing are merely by chance. This strengthens your confidence in the validity of your findings, allowing you to make informed decisions based on solid evidence rather than luck alone.
Type I and Type II Errors
Type I errors are similar to falsely sounding the alarm — like crying wolf when there’s no wolf. Picture this: you conclude that a new ad campaign significantly outperforms the previous one, only to realize later that the apparent improvement was merely a fluke. This misstep could lead to unnecessary changes that don’t actually enhance campaign performance.
Conversely, Type II errors are similar to missing the forest for the trees. For instance, you might fail to detect a significant difference in email open rates between two variations of a newsletter, dismissing the subtle but impactful changes. As a result, you overlook valuable insights that could have led to refining your campaign strategy for better results.
Significance Level
Setting the significance level is simply establishing a threshold for confidence before declaring a result real and reliable. Imagine you’re running an A/B test on two different customer journeys to see which one generates more subscribers. You set the significance level at 0.05, meaning you’re willing to accept a 5% chance of making a Type I error (incorrectly concluding there’s a difference when there isn’t). Now, if you set the bar too low, say at 0.10, you might mistakenly identify a winner when there isn’t really one, leading you to implement changes that aren’t effective. Conversely, if you set the bar too high, say at 0.01, you might overlook genuine improvements and fail to capitalize on opportunities for enhancing your campaign’s performance. So, finding the right balance in your significance level ensures you make informed decisions without jumping to conclusions too hastily or missing out on valuable insights.
The significance level and p-value work together to establish statistical significance. The significance level serves as a predetermined threshold, defining the maximum acceptable risk of falsely declaring a result as significant. On the other hand, the p-value quantifies the probability that the observed differences are solely due to random chance. Marketers compare the calculated p-value to the significance level to assess if the likelihood of chance occurrences is lower than the accepted threshold. When the p-value falls below the significance level, typically 0.05, it implies that the observed differences are unlikely to stem from chance alone, indicating statistical significance. Conversely, if the p-value surpasses the significance level, further scrutiny is warranted before drawing conclusions, as the observed differences may not be statistically significant.
Statistical Power
Statistical power refers to the likelihood of correctly detecting a true effect or difference when it exists. It’s essentially the ability of a test to distinguish between what’s real and what’s just noise. Let’s consider an example:
Imagine you’re conducting an A/B test to compare the effectiveness of two different website layouts in terms of conversion rates. You want to detect if Layout B, the new design, leads to a significant increase in conversions compared to Layout A, the control.
Now, statistical power comes into play when determining the sample size needed for your experiment. If you have a low sample size, your test may lack the power to detect small but meaningful differences between the layouts. As a result, even if Layout B does indeed lead to higher conversions, your test might fail to identify this improvement, leading to a Type II error — a false negative.
On the other hand, with a sufficiently large sample size, your test would have higher statistical power. It would be better equipped to detect even subtle improvements in conversion rates accurately. Thus, you’re more likely to correctly identify the effectiveness of Layout B, reducing the risk of missing out on valuable insights and making decisions based on inaccurate data.
Statistical power ensures that your experiment has the muscle to uncover meaningful changes, allowing you to make informed decisions that drive success in your digital marketing campaigns.
Minimum Effect Size
The minimum effect size is the smallest change that truly matters — it’s the point where we say, “This difference is practically significant enough to warrant action.”
Let’s say you’re conducting an A/B test on two different registration forms to see which one leads to more completions. You’ve determined that a minimum effect size of a 10% increase in conversion rate is meaningful to your business goals. If one layout outperforms the other by less than 10%, it might not be worth making changes based on that difference alone. However, if the difference exceeds 10%, it’s a clear signal that one layout is significantly more effective than the other.
It’s important to note that the smaller your desired minimum effect size, the larger the sample size required to achieve the set significance level and statistical power.
Armed with this understanding, marketers can focus their efforts on changes that truly move the needle and drive tangible results for their digital marketing campaigns.
Understanding the statistical concepts behind digital marketing experiments is crucial for designing reliable A/B tests and correctly interpreting the test results. Significance level, statistical power and minimum effect size directly influence the necessary sample size and should be chosen purposefully to mitigate unacceptable risks and seize pertinent opportunities tailored to each business’s unique context.
By harnessing the insights unearthed by robust A/B test findings, marketers can refine their tactics, optimize ROI and maintain a competitive edge in digital marketing. So, next time you’re designing an A/B test or analyzing your test results, remember the science behind the numbers!
Subscribe for updates
If the form doesn't render correctly, kindly disable the ad blocker on your browser and refresh the page.